In recent years, generative adversarial networks (GANs) have made significant strides in the field of machine learning, especially in the creation and manipulation of images. They've brought about new possibilities in visual content synthesis, like creating photorealistic images of faces, and landscapes, or even designing completely unique objects. However, until now, the controllability aspect of these technologies, particularly in terms of the pose, shape, expression, and layout of generated objects, has often been limited.
Many of the existing approaches for improving the controllability of GANs rely heavily on manually annotated training data or a prior 3D model. While these methods are certainly helpful, they often lack the desired flexibility, precision, and generality, hence providing restricted control to the user. It's time to bring forward a more flexible, precise, and generalizable solution to this challenge – meet DragGAN!
What is DragGAN?
DragGAN, a groundbreaking innovation in the field of image manipulation, aims to enhance user interaction and control with GANs. Its unique approach allows users to "drag" any points in an image to reach target points, thereby providing precise control over image manipulation.
The technology behind DragGAN comprises two key components:
- Feature-based motion supervision: This mechanism allows the user to drive a handle point within the image towards a target position. It provides an exceptional level of flexibility in reshaping or repositioning any part of the image.
- A point tracking approach: It uses the discriminative GAN features to continuously track the position of the handle points. This ensures precision, allowing users to achieve the exact visual results they're aiming for.
Revolutionizing Image Manipulation
Through DragGAN, anyone can deform an image with precise control over where pixels go, allowing the manipulation of the pose, shape, expression, and layout of diverse categories such as animals, cars, humans, landscapes, etc.
One of the standout benefits of DragGAN is its ability to produce realistic outputs even under challenging conditions, like creating visible content from occluded parts of an image or deforming shapes that consistently follow the object's rigidity. This capability is achieved because all manipulations are performed on the learned generative image manifold of a GAN, ensuring realism in the final output.
Qualitative and quantitative comparisons have shown the advantage of DragGAN over prior approaches in image manipulation and point-tracking tasks. It seems to be the more flexible, precise, and generalizable solution to these tasks.
Moreover, DragGAN can manipulate real images through GAN inversion, thereby expanding its applicability beyond purely synthetic content.
Looking Forward
DragGAN represents a significant leap forward in the realm of image manipulation technology. Its ability to provide an interactive, user-centric approach to GANs opens a world of new possibilities in the creation of synthetic visual content. The advent of DragGAN sets a new standard for image manipulation tasks, redefining our expectations of precision, flexibility, and generality.
We will bring more information as the field evolves. Stay tuned for more developments in this exciting field!
Read more:
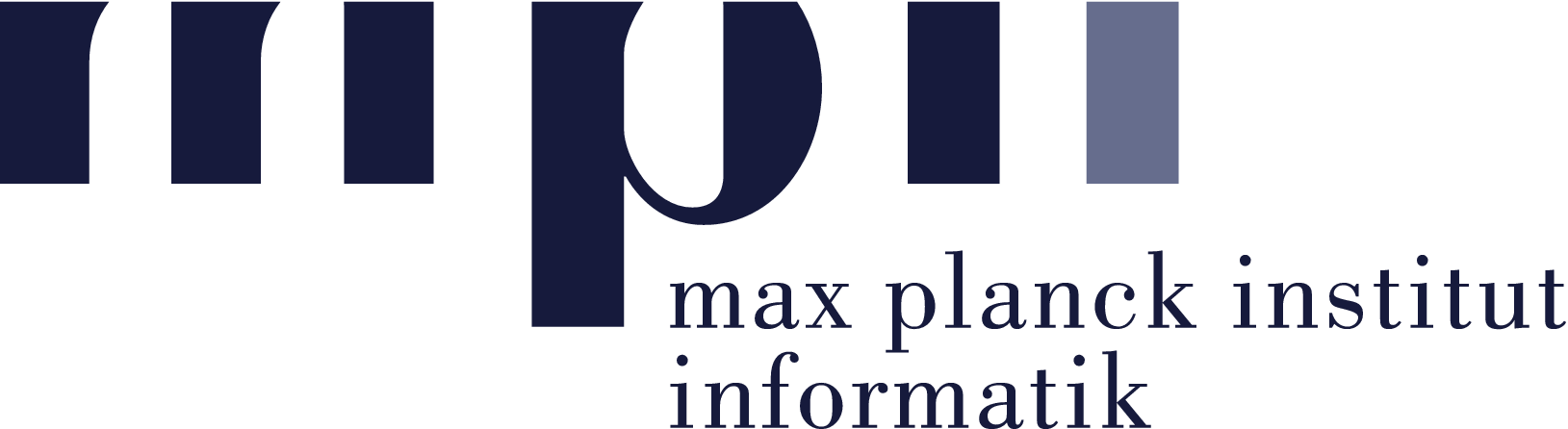
We research, curate and publish daily updates from the field of AI. Paid subscription gives you access to paid articles, a platform to build your own generative AI tools, invitations to closed events and open-source tools.
Consider becoming a paying subscriber to get the latest!